Over the past few years, the Data Mesh concept gained a lot of popularity in the data community, with large organizations talking about moving to work with it. But, what is it really, and could it save your organization?
In the following blog, Xomnia’s Lead Data Engineer Josko de Boer answers some of the frequently asked questions about Data Mesh, and tries to debunk as many of the myths that surround this increasingly popular concept as possible.
What's the difference between data lake and Data Mesh?
Data Mesh is a concept by which data is not stored centrally in one big data storage for the whole organization, but rather at the teams that generate this data. Even though it might sound simple, for many companies this approach is novel.
A data lake, on the other hand, is a large place to store all sorts of data. It is often a file/blob storage on the cloud. An organization can choose to create one big central data lake (data storage) for the whole organization.
With Data Mesh, on the other hand, we can have multiple, smaller data lakes that are hosted in different places throughout the organization. Note that Data Mesh is an architectural concept (distribution of responsibilities and tools), while a data lake is a concrete storage service found in a data platform.
What is the risk of centralizing data with data lakes?
Many organizations are finding that their central data (lake) team is increasingly becoming a bottleneck. As data becomes more central to an organization and its culture, the number of requests, use cases, and experiments starts to scale up. Meanwhile, finding skilled data employees to manage the central data lake is hard, and the costs necessary to keep it in top shape are large - and continue to grow.
One of the causes contributing to the bottleneck is the fact that central data teams spend a lot of time exploring the domain of certain products and activities within their companies They do this without directly understanding the business case and history of the IT infrastructure of that activity.
As a result, central data teams spend significant amounts of time on domain exploration without putting time into the central goal of the data team: Generating value from the data for the organization.
Don't miss Xomnia's latest business cases and blogs. Subscribe to our newsletter.
What is Data Mesh, and what are its advantages?
The term Data Mesh was coined by Zhamak Dehghani in 2019. The Data Mesh strategy doesn’t involve a ready-made architecture, but is more of a philosophy or a way of building up companies and the way they think about their data as a resource.
Data Mesh is based on four fundamental principles:
- Principle of domain ownership: The ownership, responsibility and domain knowledge of data lay with the product/activity team (the domain team). This means that analytical and operational data ownership is moved away from the data team towards the domain team.
- Principle of data as a product: Data is a product of each domain team. This means that the domain team is responsible for the analytical requirements of other teams, and is in charge of providing high quality data.
- Principle of self-serve data infrastructure platform: The data team focuses on creating a platform. This represents a central way of doing things and tooling so that the domain teams can easily take ownership of their data.
- Principle of federated governance: The interoperability of all data products requires standardizing them, a.k.a. centralizing terminology and data access. This covers the requirements of certifications like ISO or laws like GDPR. Governance is centralized for interoperability, documentation, security, privacy and compliance.
The principles of Data Mesh sound appealing for many reasons. Not only can the data team focus on their main goal, but also the people that know their domain are now in charge of that data and analytics, and are responsible for them. Below is an example that further demonstrates this:
Imagine that you work for a large retailer. The retailer has many business units focusing on different groups of products: Smart home, kitchen appliances, living room furniture, etc.
The primary sales statistics of each team are published by the respective domain teams, using the data platform tooling provided. When the smart home team is considering if they should expand into a coffee table with a built-in speaker, they can easily check how the coffee table sales are doing. But, they run into a small issue - the profit margin isn’t clear. They simply talk to the kitchen furniture domain team and the new information is added to their data product. The smart home team is now able to evaluate the business case for smart speaker coffee tables.
This contrasts heavily with the centralized data lake approach. The data lake takes all data into a repository of data in its natural format. It usually has several zones (e.g. bronze/silver/gold) of data that has been checked for quality, issues and consistency. In that approach, a central team is responsible for ingesting, transforming, enriching and publishing all data. That team lacks the domain knowledge to do this properly, usually leading to quality issues downstream. Simply put, no team can be a domain expert on all data in a (large) organization.
Even though the second scenario represents a good system that works in many setups, it lags behind the first scenario thanks to Data Mesh: Decentralized data with good tooling and a responsibility that lies with people who know the domain.
All this makes Data Mesh sound like a dream come true. But what about the other side of the coin?
Join our team of top-talent data and AI professionals. Click here to view our vacancies.
What are the disadvantages of Data Mesh?
Data Mesh isn’t a one-size-fits all solution that any company can employ. In the sections below, I list a few downsides of Data Mesh that explain why it is not the best option for all companies.
1) High transformation costs
Big organizations with years-old data teams should be particularly weary ahead of the decision to move to Data Mesh. In fact, it is not a surprise if Data Mesh isn’t a good option for them at all. This is because as you move into the Data Mesh, you invest a lot of time into the data platform architecture, and might find that it can’t be applied to every team.
Here is an example:
Your organization has been around for a while. Each team has a scala of software tools that consists of a mixture of custom solutions built internally and useful external services.
It is unlikely that the domain team actually already knows what their data looks like. They will have used the metrics and functionality provided by the tools they have, which they most probably didn’t build themselves. You could have someone from the central team join them, or you could hire an experienced consultant, who will take some time to onboard them and help them out.
Let’s say you lend them a data scientist or analyst to check their data with them, and hire someone with more technical expertise after that. Diving into the current tooling, it is likely that you will run into historical technical debt in the current tooling (because, let’s face it, we always do). You might find tables that are joined sloppily, quality issues that were never clear before, or simply that you can’t convert KPIs from one team to another - despite the federated governance team setting up clear definitions of what will be used in the company.
This is a hard thing to solve. You will have to pull in each team separately, which is a political adventure next to being a technical exercise. Domain teams will have to become mature owners of their own data and insights, and monitor quality for other teams. This whole process will consume time and resources that might not be as economical as other less fundamental solutions.
2) Organization and ownership challenges
Assuming you went down the path to transform to Data Mesh, you have to convince each domain team that they are now the owner of their data. Pushing back and trying to get them to take their ownership is a minefield - not only is the data now not available, but also other teams are depending on the broken team’s data product.
If their data pipeline fails, they are inclined and used to contacting the data (platform) team or software engineering teams to fix the issue. However, the boundaries of that system have changed - domain teams are in charge of data, so if the problem doesn’t lie with the data platform tooling, they should fix it themselves. In reality, however, it is very likely that each team will initially escalate each problem. The tool didn’t work, so the tooling they were given must be at fault, right?
The issues keep coming in for the source data team. Meanwhile, other teams hear that the data platform is broken and that it won’t be fixed. Suddenly, the data platform team is seen as the responsible one. You tell them it’s the domain team that owns the data, but they deflected it to you and the dependent team is not buying it. They escalate and a higher level of management gets involved…
That doesn’t sound great. The only way to prevent this is to make sure the domain team knows that they are the owner, and are capable of evaluating the error and whether this is something that remains within their ownership, or needs to be escalated to you.
In a nutshell, big organizations that have multiple teams need to carefully calculate the cost and repercussions of implementing Data Mesh both culturally and organizationally. They shouldn’t be scared to admit if the costs of such transformation outweigh its merits.
3) Data without boundaries or clear ownership
Companies need to be mature enough when it comes to data ownership before deciding to adopt Data Mesh. If your company isn’t mature enough, the transition to Data Mesh might be costly, cumbersome, and even dangerous (in the case of sensitive data).
Suppose your team is in charge of creating data about certain company assets. Another team is working on a UI that displays the current location of assets and wants to poll your API to get the right metadata about the asset. In a mature mesh organization, you can depend on that team to treat the data properly and securely. If you tag fields as sensitive, you are sure the other mature team will take care of that.
But what if your organization isn’t mature? As the owner of that data, you set up a process to evaluate if the proposed setup of the other team meets your requirements for the data you own. This leads to a set of forms, meetings, a paper trial and just generally a slow process.
Another important question is how to treat sensitive data (e.g. personal information) when it transitions boundaries? To make sure that this will not lead to issues, teams will build elaborate walls and paper trials to protect themselves from negative consequences. This will, in turn, slow down processes involving the data.
Here’s another ownership issue, and one that is even harder to solve: Suppose you have a team that is in charge of revenue, and another team that is in charge of part of the product. The product team imports the revenue data for their product and publishes that along with the product data to provide insights into the monetary value. Who is now the owner?
Anyone consuming the data of the product team will now assume that that team owns it, but the data is coming from another team. What’s more, there will be business logic in place to combine the data from these two different parts of your mesh. That logic confounds the issue - who owns the business logic, and if errors arise, what domain team is at fault?
This increases in complexity almost infinitely as data moves from consumer to producer and onward, crossing boundaries in the mesh and team ownerships. Who is to say whose business rules are at fault?
Achieve your organization's full potential with the leading AI consultancy in the Netherlands
Which organizations are best fitted for Data Mesh?
Having read all my concerns and criticism, you might think that I am opposed to the Data Mesh. This isn’t entirely true - I am against adopting it at the moment, considering the current level of maturity in most companies. I am also against adapting it in every available kind of organization.
There is one kind of organization that seems perfect for Data Mesh: Teal organizations. These organizations are already based on fully autonomous cells, with former management and staff taking the role of a coach or mediator. They focus heavily on onboarding, company culture and way of working. Each team contains the roles, qualities and responsibilities so that they can act autonomously.
As a result, I think teal organizations are a perfect fit for Data Mesh, because the thought processes there perfectly mirrors those of Data Mesh.
The Teal organizations are based on the following principles:
- The need for autonomy,
- self-organization,
- making sure people are sufficiently competent at the way of working, and
- the centralization of some exports to provide a central platform. This is because in teal organizations there is usually a central group of couches and HR specialists. In Data Mesh, this is mirrored by having a data platform team.
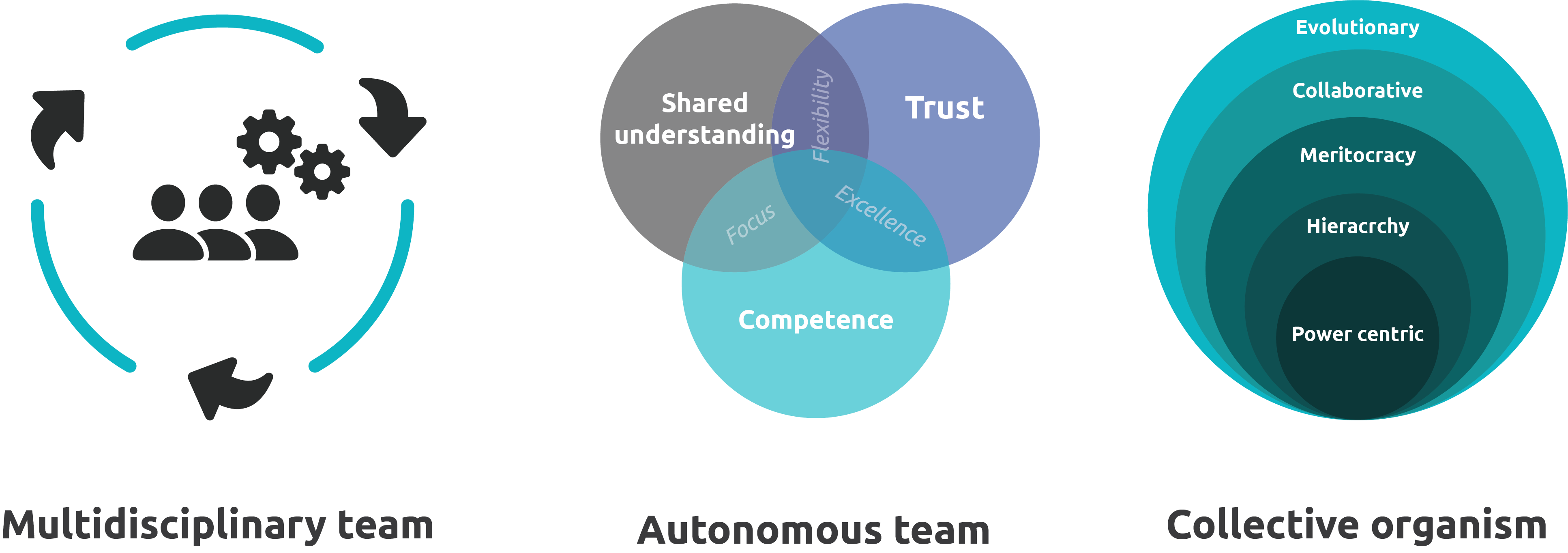
What are the synergies between teal organizations and Data Mesh?
The change to teal organizations involves very similar concerns. You can’t just decide to go teal - you need to carefully change the management, train people in the new way of working, etc.
Companies using teal organization structure exist - in fact the book that defined teal goes into many examples. Those examples encompass any size and any sector, in the commercial and government/non-profit sectors.
One thing these organizations usually share is a large focus on coaching different teams (similar to a mesh cell), heavy onboarding processes and making sure people understand and are happy with that culture.
So let’s reconsider from the perspective of teal organizations the situation whereby team A takes the data of team B and does something to it. Consider a situation where team B did something to the data and now team A is being blamed for something they didn’t do. This is now a conflict situation.
In the teal setup, both teams would have had extensive training on conflict resolution. Likewise, team A and B now have to resolve their conflict. They enter a meeting according to company policy (Let’s assume there’s some form of Non-Violent Communication in place).
Team A starts the meeting. “Hey Team B, we see that you have done [something] to the data. This led to an unfortunate issue where we were blamed for the issue. This made us feel fairly frustrated. What we need from you is to take ownership of the data that you publish after enriching it. Do you think you could do that?”
Team B responds: “Hey team A, I hear you. Sadly we were missing a piece of documentation and we thought that this particular piece of data left our cell without editing. We’re very sorry! We have taken steps to improve documentation and will now take ownership of this part of the pipeline.”
Sounds easy enough, right? But this kind of process only works if all teams are mature, or in other words, if your organization is mature when it comes to data. And that usually requires a lot of change management - and change is hard.
To Data Mesh or not to Data Mesh? Here is the verdict
I think Data Mesh is a good fit for some companies. Even though the move towards it is complex and needs careful thought and consideration, it still sounds better, is more fun to work with, and puts people's skills and diversity to better use.
Having said that, I do not think Data Mesh is a logical step in the digital transformation of every organization, especially the more established and slower moving ones. Simply put, when you’re used to waterfall planning, isolated teams and long-term planning, Data Mesh probably isn’t for you.
But what if you are already an agile organization at the end of most of your digital transformation with very mature cross-functional scrum teams? Then you might be able to make the jump. This will require training and onboarding to keep up with the dramatical change of course, but it can be done.
What about startups that are in the setting up processes? Well, we all know that startups can be a bit chaotic. But moving towards setting up a proper Data Mesh in the startup phase and the scale up phase have definitely been done. Indeed, I think most new digital startups are an excellent fit for this kind of system, because you can implement and onboard from the start. You don’t need to do much change management - you are starting off this way. It’s your strategy from the ground up.
So, Data Mesh: Yay, but the journey towards it is usually a Nay.