This blog is the second in a series on AI business challenges, written by Xomnia’s analytics translators.
Running and completing a marathon is a major achievement. It takes months of dedicated training, investment in the right gear and an adjustment of your lifestyle to finish it. The achievement is so admirable that many people take a shot at making it. Some try year after year to reach their goal. However, almost no one would invest in expensive gear, hire a trainer and then try to finish a marathon in the next week.
This is exactly what often happens in the world of data and analytics: many organizations start investing in data science without having a foundation in place, and expect results within six months.
Data and AI managers today have a challenging task: to figure out how their organizations are going to achieve the ambition of finishing a marathon towards data maturity. This blog goes in depth on how to ensure that your organization finishes the marathon in a sustainable way.
Download Xomnia's Way of Working whitepaper
Start with an AI strategy
Having an AI strategy in place is a good way to determine what data (science) products may help your organization, and how they are to create value. It is crucial that an organization is willing to invest time and resources to explore and exploit the opportunities that data and AI have to offer.
An AI strategy should describe an organization's ambition and desired state in relation to the technology. Spotify, for example, uses data to provide a lifetime of content for its users (Google Cloud Summit, 2021). They rely on machine learning techniques at the core of their business to ensure that customers keep listening to content on their platform. To read more on creating an AI strategy, you can visit a previous blog that discusses this topic in depth: Demystifying AI strategy: Xomnia’s AI value proposition method. The diagram below summarizes how an AI strategy is formulated:
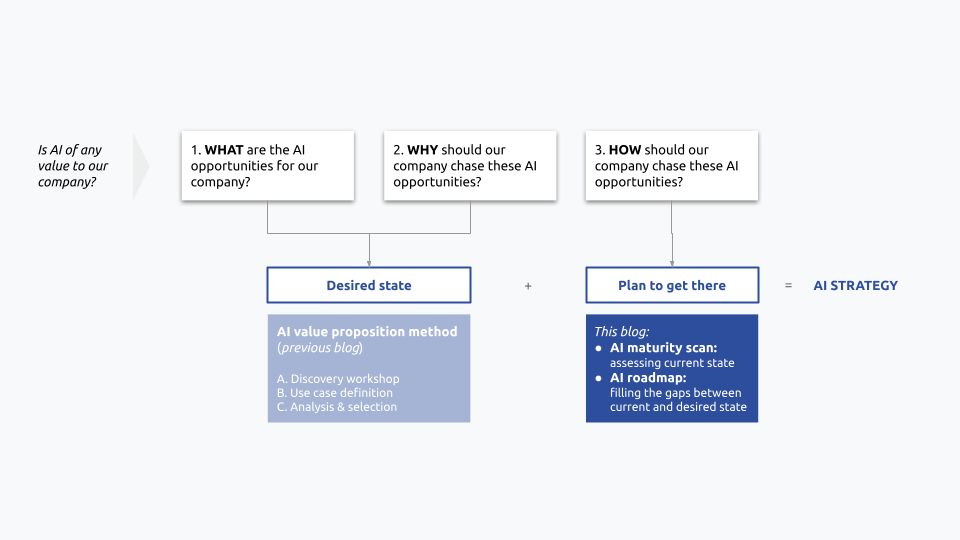
Determining the current state of AI in your organization
Achieving the desired state requires an organization to execute one or more project initiatives, like building a data platform to support advanced data products. The gap between the desired and current state of AI maturity within the organization determines the size and impact of the required projects. The projects will also have a dependency between each other.
To assess the current state of data and AI maturity of the organization, a maturity framework can be used. In the past years, several frameworks have been published, for example by Dell, Gartner (2012), Snowplow Analytics, Datacamp and probably many more. The goal of these frameworks is to provide guidance to determine where the data and AI capabilities within an organization stand. Most frameworks mention similar aspects, like ‘governance’, ‘people’ and ‘technologies’.
Data (product) managers will recognize these data maturity frameworks, as they are often used to support product teams to focus on the right products and activities. Instead of using the frameworks to analyze your entire organization, we recommend focusing solely on the identified value propositions and problems within your organization. If your AI strategy focuses on operations for example, it should be ensured that solutions can be developed and implemented in that area. Managers should therefore assess the relevant aspects within that area in the data maturity framework to determine how to achieve the desired state.
Finally, relating the current state to the desired state enables a purpose driven roadmap with clear priorities and focus.
Complete Xomnia's free Data Maturity Self-Assessment
Example: Assessing your AI maturity
Assume that you are the data science and analytics manager of a large organization that sells different products to customers. In the past period, the small team of business intelligence analysts has successfully created insights to help your business understand the customers and their performance. Data and AI are getting a more prominent place in the business strategy as C-level management sees the potential to outperform competitors in the market with hyper personalized offerings.
The example describes a desired state and also gives some indication of the current state. To do a proper analysis of the state of data maturity within an organization, we consider four aspects to determine what is needed to achieve our desired state:
- People
- Technology
- Data
- Governance (Process)
In this analysis, we focus on the state within the marketing and digital department, as these are the departments where our solutions will be implemented.
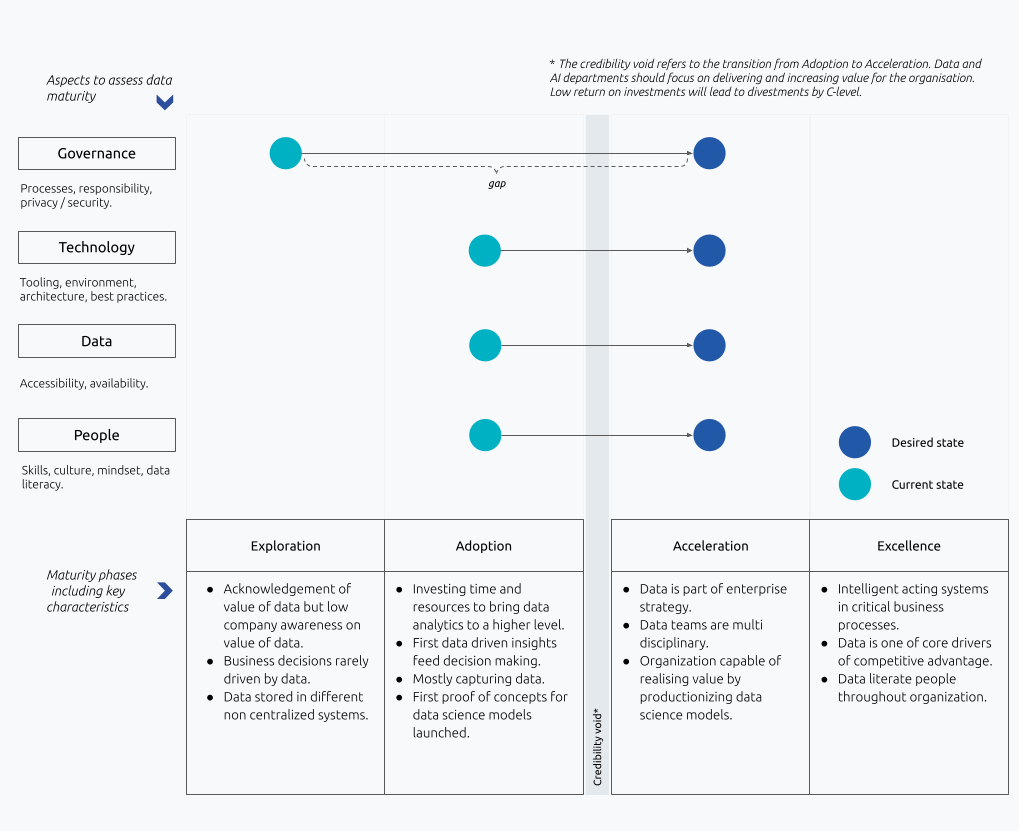
As shown by the diagram above, by identifying the gaps on predetermined aspects, we notice that both on an organizational and a product team level several things have to be brought in place. In addition, data teams also have to prove to the organization that data and AI solutions can actually live up to the promise.
- People
To offer hyper personalized offerings to customers, the organization will need to attract data scientists, data engineers and product owners to develop and deploy complex machine learning solutions. A new team has to be created with the relevant skills that BI analysts alone, for instance, cannot provide. Without these expertise, it will not be possible to develop and deploy the necessary solution. - Technology
The organization has a business intelligence environment in place. To develop more advanced data products, more advanced tooling is required. Tools like Tableau and PowerBI are useful to look back and investigate, but not suitable to make predictions. Data scientists will need to have access to tools like Databricks or Jupyterhub to develop the solutions. In addition, they might also need other runtimes, like GPU resources. Data engineers will need to be able to use workflow orchestrators (e.g. Airflow), container software (e.g. Docker) and CI/CD (e.g. Jenkins). - Data
Complex and advanced data products, for example those based on machine learning algorithms or real time data processing, have different requirements than creating insights. Initiatives have to be identified and prioritized to realize hyper personalized offerings. - Governance (Process)
Allowing a machine learning algorithm to determine which customer gets which offering, without human intervention, is thrilling. It requires confidence and trust, but every organization also needs to figure out the governance. Who is responsible for the outcomes and effects? Is the solution biased and who is responsible for investigating that? How to handle maintenance of the solution? The organization will have to ensure that crucial questions are answered, before the solution starts creating offers for customers. Governance is especially important for organizations that move from BI use cases to more advanced use cases. If the hyper personalized offering is deployed but results are senseless, this can have serious consequences on your organization.
Download Xomnia's Way of Working whitepaper
Bridging gaps: the AI roadmap
With a clear understanding of the gaps on different aspects in the data and AI maturity, we must plan our actions to bridge them. The gaps that we have identified can be translated to initiatives. Initiatives are the foundation for practical problems and/or opportunities that can be assigned to product owners and development teams to start exploring and executing. The ideal format to do this is an AI roadmap.
A data and AI roadmap can be used to communicate progress within an organization. Data and AI managers should update the document periodically and focus on the most important initiatives, ones with dependencies with other projects, to be brought in place. This also allows them to create buy-in and align with relevant teams to ensure resources are available when they are needed. The data and AI roadmap should act as guidance and not as an ultimate truth over the course of several months/years. Assumptions are often proven to be wrong. You can read more about the execution in our whitepaper on successfully developing data products.
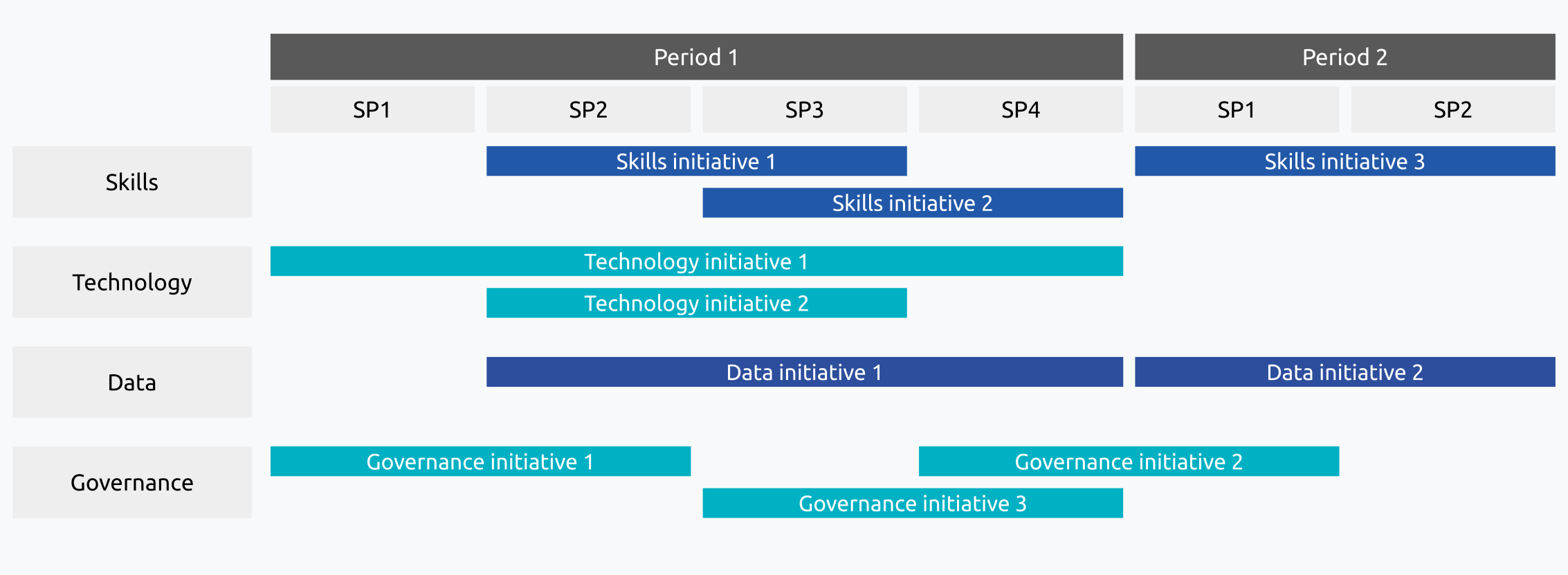
Complete Xomnia's free Data Maturity Self-Assessment
Sustainable steps to achieving your desired AI maturity state
To run a marathon, we also have to compare where we are standing today with where we want to be. Our level of fitness probably needs to improve drastically, equipment should be bought and be practiced with and shorter distances (5k, 10k, 20k) should be achieved to be confident enough to run the full 42,195 km.
Finally, To keep up your good spirits, celebrating the accomplishment of intermediate milestones is as important as celebrating “the big one”, as it signals your progress in comparison to your prior performance. Identifying the right gaps and prioritizing the actions to overcome these gaps will significantly increase the likelihood of success.
---
Xomnia has a team of analytics translators (ATs) dedicated to assisting clients with the business aspects of AI challenges. They do so in several roles, such as AI product owner (product-focused), AI evangelist (adoption-focused), AI ethicist (responsibility-focused), or Strategic AI advisor (strategy-focused). In these series of blogs our analytics translators give an insight into their work. Blog one was focused on AI strategy. In the upcoming months blogs will be published about how to define the right use case/initiative, how to generate value with data products and incorporating responsible AI. If you are interested in how to successfully develop a data product. You can also download our whitepaper on this topic.
Would you like to learn more or have a conversation about AI business challenges at your organization? Get in touch: info@xomnia.com.