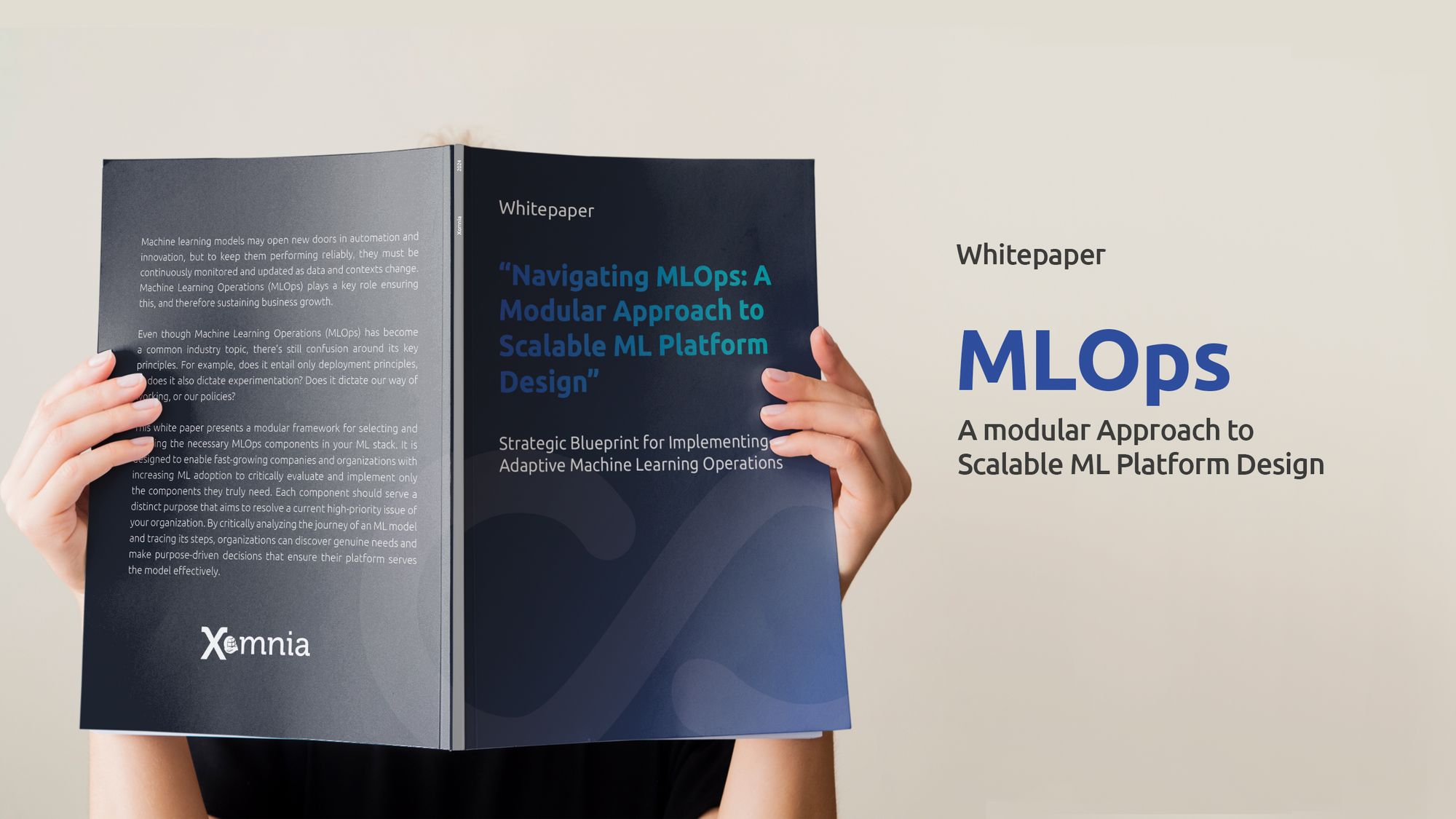
Even though Machine Learning Operations (MLOps) has become a common industry topic, there’s still confusion around its key principles. For example, does it entail only deployment principles, or does it also dictate experimentation? Does it dictate our way of working, or our policies?
Unlock the potential of your ML stack with our modular MLOps framework. Tailored for fast-growing companies, this guide helps you select and implement only the components you need to tackle high-priority challenges. By analyzing the journey of your ML models, you can make informed decisions that optimize your platform's effectiveness. Start building a purpose-driven ML strategy today!
What is MLOps and what does it?
With the rapid rise of AI and ML, companies need structured methods to deploy and manage ML solutions effectively. MLOps is a set of best practices for operationalizing machine learning models at scale. At Xomnia, we believe MLOps is not only about the technology itself, but also about how it is applied- encompassing processes, policies, and organizational considerations.
Modern machine learning platforms are composed of components that align with different stages of the ML model lifecycle— Development, Deployment, and Monitoring. Each component serves a specific function, ensuring that the platform is both efficient and practical. Importantly, MLOps drives efficiency, scalability, and faster time-to-value by standardizing processes and automating workflows. Enhanced traceability improves collaboration, while proactive monitoring ensures reliability and cost saving. These benefits unlock ML’s full potential, delivering impactful insights that align with business goals.
The best practices around MLOps align with the steps of the machine learning model lifecycle, focusing on the need to automate or make each step more efficient. With this definition, it provides the framework for designing ML platforms with purpose-driven components that support model development, deployment, and monitoring.
What is the current challenge in how teams approach MLOps
Organizations face numerous challenges when implementing machine learning at scale, including fragmented tools, lack of reproducibility, and limited insights into model performance. These hurdles make scaling ML efforts both complex and inefficient. MLOps is often seen as the “holy grail” for addressing these issues, promising to streamline and standardize the ML lifecycle.
The reality, however, is that no one fully agrees on what MLOps is and what it truly entails. Is it a framework, a set of technologies, a process or the lifecycle of a model? This lack of consensus leaves organizations uncertain of where to start with implementing MLOps, resulting in disjointed efforts that fail to deliver meaningful results.
We advocate for a structured MLOps approach that starts with a clear purpose for each component. Instead of choosing technology first, this white paper encourages organizations to address business needs first, then identify the best process to serve that purpose, and finally select the appropriate tools.
A structured MLOps approach ensures organizations focus on solving specific business problems rather than building bloated platforms with unused components. By aligning solutions with business needs and user-centric design, companies prevent wasted effort, maintain focus, and drive adoption. This approach streamlines workflows, maximizes platform utility, and ensures long-term success. Download our white paper to read more.